ISG Winter Meeting 2023
Themed Oral Presentations - Hepatology and Other GI
First Prize
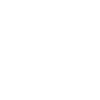
Dr John Campion
Mater Misericordiae University
Large Language Model Chat GPT-4 Can Outperform Clinicians in Endoscopy Triage
TBA (23W160)
Large Language Model Chat GPT-4 Can Outperform Clinicians in Endoscopy Triage
Author(s)
J R Campion1,2, J Cudmore1,2, E Keating1,2, H Kerr1, J Leyden1,2, B Kelleher1,2, J Mulsow1,2, C Lahiff1,2, G Bennett1,2
Department(s)/Institutions
1. Department of Gastroenterology, Mater Misericordiae University Hospital 2. School of Medicine, University College Dublin.
Introduction
Large language models (LLMs) such as Chat GPT-4 utilise machine learning techniques to generate answers to queries, and could be harnessed to assist with the clerical burden that takes clinical staff away from front line duties.
Aims/Background
We sought to compare adherence to current national and international guidelines on triage and surveillance endoscopy between Chat GPT-4 and gastroenterology staff.
Method
64 fictional patient cases were generated by referencing national and international guidelines. The cases were divided across five categories: lower gastrointestinal symptomatic (LGI), upper GI symptomatic (UGI), family history of colorectal carcinoma (FHCC), polyp surveillance (PS) and Barrett’s oesophagus surveillance (BS). Clinicians (doctors and nurses) and Chat GPT-4 were asked to triage the cases from memory (attempt 1), and again when given the relevant guideline for reference (attempt 2).
Results
20 clinicians and one LLM participated in the study. In attempt 1, the LLM median (IQR) score was higher than clinician in LGI [70 (60,70) vs 50 (37.5,60), p=0.008] and FHCC [82 (73,82) vs 36 (27,65), p = 0.003] while there was no statistically significant difference in BS [71 (64,71) vs 57 (43,64), p = 0.37)], PS [31 (23,46) vs 31 (21,48), p=0.84] or UGI [50 (50,62.5) vs 53 (50,57), p=0.81)]. In attempt 2, median clinician scores improved for LGI [80% (70,80)], FHCC [78% (52,91)], BS [79% (67,86)], PS [62% (42,71) and UGI [75% (74,81)].
Conclusions
LLMs may prove a useful tool for specific healthcare tasks but unsupervised decision-making cannot yet be delegated to LLMs. Specific models trained only on discrete inputs such as relevant guidelines may improve performance and become a reliable adjunct to conventional healthcare processes in the future.